Intelligent Self-Learning Sensors with Online Real Time Calibration
This project designs and tests innovative millimeter-scale low-cost environmental monitoring sensors, focussing on sensor suites that have the largest impact on occupant comfort and building energy efficiency as well as sensors having numerous downstream applications such as data-driven control of ACMV systems, situational awareness provision, and real-time integration of occupant preferences.
This project studies several innovative research directions such as aggressive, low-voltage power management, energy harvesters, and reconfigurable on-chip power conversion circuits. Energy quality scalability will be realized through on-chip analog/ digital components that reduce the energy per measurement when coarser quantization or sampling rates are adequate.
This project also studies novel sensor node architectures to gain unprecedented on-chip ability to continuously learn from physical data and identify behavioral patterns locally. This approach creates new opportunities to (a) drastically reduce significant energy consumption associated with radio-frequency transmission, and (b) digitally compensate analog imperfections by incorporating them into the learning process, thus substantially reducing energy and silicon area (i.e., cost) of the sensor node.
This project will also address the challenge of maintaining performance in distributed sensor networks over their extended operational lifetime. The research enables in-field non-intrusive continuous calibration, which is a radical departure from the traditional approach to tolerate faults through sensor redundancy. Statistics on sensor quality and battery state-of-charge drive Bayesian inference algorithms will be used to construct optimal estimates of the sensed spatial field.
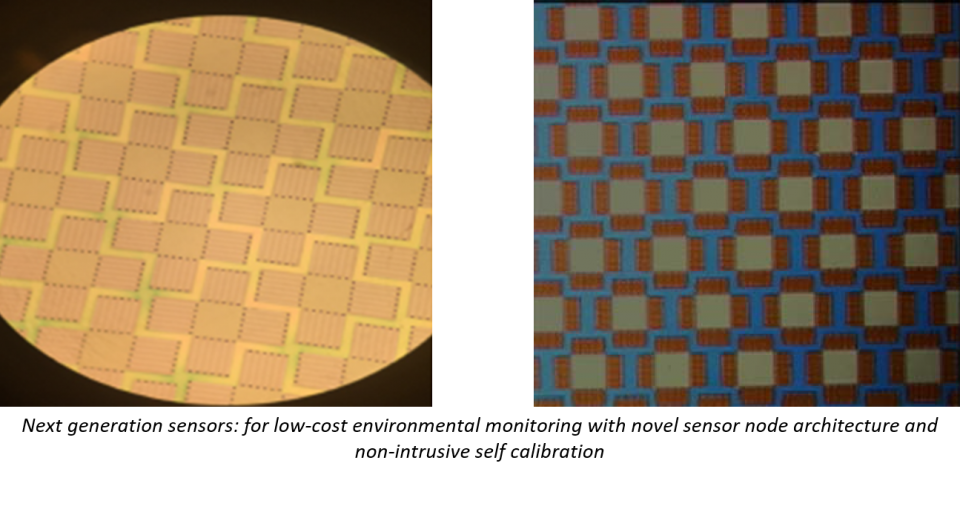